The insurance industry is changing fast. It relies on data and tricky risk calculations. Now, Artificial Intelligence (AI) and Machine Learning (ML) are driving this transformation. These technologies are changing many parts of the industry. They impact customer engagement, underwriting, claims processing, and risk assessment. AI and ML bring automation, efficiency, and precision. They help insurance companies offer tailored services. They also detect fraud and enhance operations like never before.
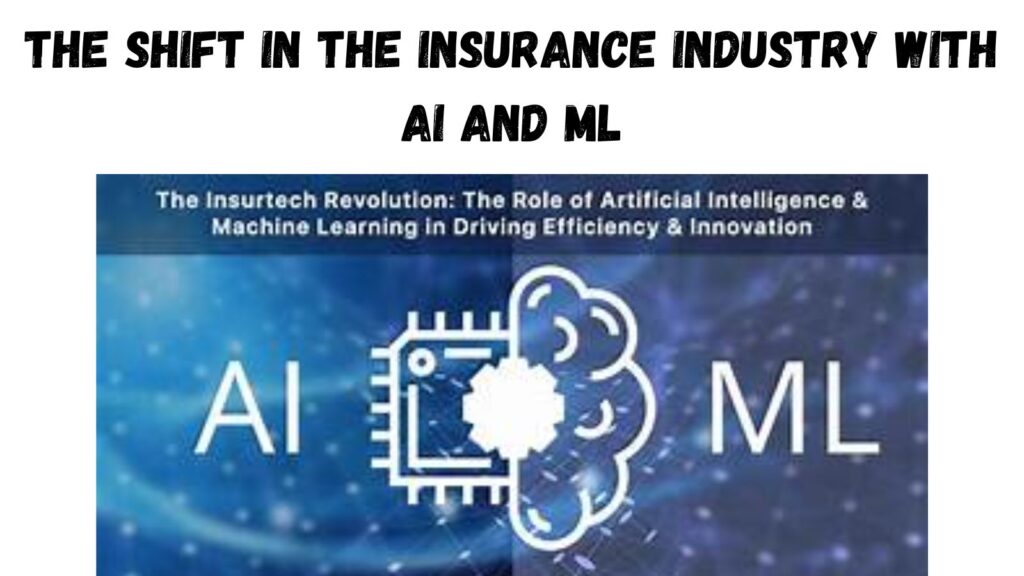
As AI and ML technologies grow in the insurance sector, they bring important concerns. One key issue is testing standards. These technologies offer great benefits. However, their rapid adoption has exposed major gaps in testing protocols. These gaps can lead to inefficiencies, errors, and even compliance risks. Testing frameworks and standards are key. They help make sure AI decisions are reliable, clear, and fair. This is especially important in regulated and consumer-focused fields like insurance.
The Need for Robust AI and ML Testing in Insurance
Contents
- 1 The Need for Robust AI and ML Testing in Insurance
- 2 Chandra Shekhar Pareek: Bridging AI and ML Testing Gaps
- 3 Bridging AI Testing Gaps in the Insurance Industry
- 4 Real-Time Examples and Impact
- 4.1 Fun Fact: The History of AI in Insurance
- 4.2 Conclusion: The Future of AI and ML in Insurance
- 4.3 FAQs
- 4.4 Why is AI and ML testing important in insurance?
- 4.5 What are some common issues in AI models for insurance?
- 4.6 How does Chandra Shekhar Pareek address bias in AI models?
- 4.7 What is explainable AI (XAI), and why is it important?
- 4.8 How can AI testing improve risk-based decision-making in insurance?
Testing is crucial in AI and ML systems, especially in insurance. Before we look at specific standards, let’s explore why this is important. AI and ML are data-driven technologies that rely on large datasets to make predictions and decisions. In the insurance industry, these systems are used for tasks such as:
- Risk Assessment: AI algorithms look at large data sets to find risk factors. This helps insurers set competitive prices and spot high-risk individuals or businesses.
- Underwriting: Machine learning models help insurers predict claim likelihood using past data. This leads to quicker and more accurate decisions.
- Claims Processing: AI speeds up claims by automating the process. This cuts down on settlement time and boosts payout accuracy.
- Customer Engagement: AI chatbots and virtual assistants boost customer service. They offer instant help and tailored interactions.
While these applications hold great promise, they also come with risks. A poorly tested AI system can lead to biased results. It might not follow regulations and could create inefficiencies. These issues can reduce the advantages of automation. Strong testing is vital. Without it, AI decisions can lead to financial losses, regulatory fines, and damage to customer trust. An AI model that misjudges risk can cause problems. If it underprices, the insurer faces more claims. If it overprices, customers might leave.
The answer is to create and follow strict AI and ML testing standards. Industry expert Chandra Shekhar Pareek is working hard on this.
Chandra Shekhar Pareek: Bridging AI and ML Testing Gaps
Chandra Shekhar Pareek is a prominent figure in the field of AI and software testing. Pareek holds certifications such as the AI Certified Test Professional and ISTQB. He has earned a strong reputation for creating testing methods that tackle the unique challenges of AI and ML systems. He has strong skills in software testing and life insurance. He also knows a lot about regulatory compliance and customer service. His LOMA certifications (ALMI, FLMI, AIRC, ACS, and ARA) show his expertise.
Pareek’s work focuses on two key areas in AI and ML testing within the insurance industry:
- AI Model Explainability: Pareek’s key contribution is creating methods to test AI models. These methods make sure the models are clear and understandable. In the highly regulated insurance industry, it’s not enough for AI systems to make accurate decisions—they must also be transparent. Stakeholders, like regulators and policyholders, need to know how decisions are made. This is important, especially when those decisions affect pricing, claims, and underwriting. Pareek’s focus on explainability helps insurers gain trust from customers and regulators.
- Risk-Based Decision-Making: Pareek focuses on making AI systems’ risk-based decisions more reliable. Pareek has helped insurers reduce mistakes in underwriting and claims processing. He does this by using strict testing standards. It boosts operational efficiency, lowers risks, and builds trust with policyholders. By checking how well AI models work, Pareek has helped insurers cut costs. This also improves the quality of their services.
Bridging AI Testing Gaps in the Insurance Industry
To grasp why it’s vital to bridge AI and ML testing gaps in insurance, let’s explore the challenges and how Pareek’s methods tackle them.
1. Bias in AI Models
AI and ML systems often use historical data for training. This can lead to the unintentional spread of existing biases in that data. If an AI model learns from data showing biased hiring or unfair claims, it may make unfair underwriting choices. These decisions can harm specific groups.
Chandra Shekhar Pareek’s approach to AI testing emphasizes bias detection and mitigation. He uses advanced testing frameworks to check AI systems for fairness and equity. This ensures that models do not unfairly impact protected groups. Insurers can avoid regulatory problems and lawsuits about discrimination by doing these tests.
2. Model Drift and Validation
As AI systems are exposed to new data, they can evolve or “drift,” causing their predictions to become less accurate over time. This is a big issue in insurance. Accurate risk assessments are key for making profits and following regulations.
Pareek’s testing methodologies include model drift detection and continuous model validation. Insurers can keep their risk assessments accurate and reliable by regularly testing AI systems. They should also update these systems with fresh, relevant data. This helps minimize the chance of financial loss.
3. Lack of Transparency in AI Systems
Many AI models in insurance, like deep learning neural networks, are called “black boxes.” They work in ways that are hard to grasp. In insurance, transparency is essential to meet regulatory requirements and build customer trust.
Pareek’s focus on AI model explainability has helped fill this gap. He supports using explainable AI (XAI) techniques. These help stakeholders see how AI makes decisions. For example, if an AI system denies a claim or increases a premium, it’s essential for both the insurer and the customer to know why the decision was made. Pareek’s methods help ensure that AI models can be audited and explained in simple terms.
4. Compliance Risks
Non-compliance can lead to fines, penalties, and reputational damage.
Pareek has aligned AI testing standards with regulatory frameworks. This helps insurers ensure their models follow laws like the General Data Protection Regulation (GDPR) in Europe and Fair Lending Laws in the U.S. Pareek uses strict testing methods. This helps insurers steer clear of legal problems and keep high customer protection standards.
Real-Time Examples and Impact
Let’s look at some real-time examples of how AI and ML testing gaps are being bridged in the insurance industry:
- Claims Processing: A big insurer used an AI claims processing system. However, they struggled to keep the model’s decisions accurate and clear. Using Pareek’s AI testing framework, the insurer cut errors in claims assessments. This change also boosted customer satisfaction by offering clearer reasons for claim denials.
- Underwriting Accuracy: Another insurer utilized an AI model to assess underwriting risks. However, the model’s predictions were frequently inaccurate due to model drift. The insurer enhanced its underwriting process by using Pareek’s techniques for continuous validation and drift detection. This also led to a reduction in rejected policies.
- Customer Engagement: A top insurance company used an AI chatbot for customer service. However, it faced problems with bias in its replies. Pareek’s bias detection and mitigation techniques helped the chatbot treat all customers fairly. This approach boosted both engagement and trust.
Fun Fact: The History of AI in Insurance
AI has been in insurance for decades. But in recent years, big data and advanced machine learning have unlocked its true potential. One interesting fact is that early AI applications in insurance were focused on fraud detection. The first AI fraud detection system came out in the
Conclusion: The Future of AI and ML in Insurance
AI and ML are changing the insurance industry. However, without strong testing standards, there are big risks. These include inefficiencies, bias, and non-compliance. Chandra Shekhar Pareek helps insurers manage the challenges of AI and ML. His work focuses on trust, transparency, and following regulations. His contributions are key. They help make sure AI decisions in insurance are accurate, efficient, fair, and clear.
As the insurance industry continues to evolve with AI and ML, it is crucial that insurers adopt and adhere to rigorous testing standards. By doing so, they can unlock the full potential of these technologies while safeguarding against the risks they may pose.
Louisiana’s Auto Insurance Crisis and Gov. Jeff Landry’s Legislative Agenda 2025
FAQs
Why is AI and ML testing important in insurance?
AI and ML systems play a critical role in areas like underwriting, risk assessment, and claims processing. Rigorous testing ensures that these systems are accurate, fair, and compliant with regulations.
What are some common issues in AI models for insurance?
Common issues include bias, lack of transparency, model drift, and compliance risks. These can lead to inaccurate predictions, legal issues, and customer dissatisfaction.
How does Chandra Shekhar Pareek address bias in AI models?
Pareek uses advanced testing tools to find and reduce bias in AI models. This helps ensure that decisions are fair and equal for all customers.
What is explainable AI (XAI), and why is it important?
Explainable AI (XAI) is about AI systems that clearly explain their decisions. In insurance, this helps build customer trust and ensures regulatory compliance.
How can AI testing improve risk-based decision-making in insurance?
AI testing keeps models accurate and reliable. It reduces errors in underwriting and claims processing. This process also improves risk assessment overall.